Integrating AI-powered helpdesks into your IT customer support system can be a game-changing move. It helps automate routine tasks, quickly resolves common inquiries, and gives support agents real-time, data-driven insights. This reduces response times and improves the accuracy and relevance of support. AI’s ability to learn from interactions ensures that service quality continuously improves.
AI-powered helpdesks also enhance the customer experience by offering personalized support on a large scale. Analyzing customer data and interaction history allows AI systems to tailor responses and recommendations, making the customer journey more engaging and satisfying. Hence, it increases customer satisfaction rates and strengthens loyalty and trust in the brand.
Related article: Transforming Customer Support: An Implementation Guide to Generative AI-Assisted Helpdesks
How to Implement AI-Powered Helpdesks?
Now, let’s look at a step-by-step approach to implementing AI-powered helpdesks.
Step 1: Clearly Define Objectives
When integrating AI into helpdesk operations, the initial step is to precisely define your objectives. This foundational step is critical because it sets the direction for the entire project. The goals can vary widely but typically include:
- Automating tasks that are repetitive or do not require human intervention, AI can streamline operations
- Instantly responding to customer inquiries, drastically cutting down wait times and improving the customer experience
- Contributing to higher satisfaction levels among customers
Step 2: Assess and Finalize Needs
After defining your objectives, the next step is to conduct a thorough assessment of your current helpdesk operations to identify specific pain points and areas where AI can deliver significant improvements. This assessment involves:
- Inquiry volume: Analyze the volume and types of inquiries your helpdesk receives since high volumes of repetitive questions are prime candidates for AI automation.
- Response times and backlogs: Identify any issues with response times or backlogs; AI can help manage these by providing instant responses to common queries.
- First Contact Resolution (FCR) rates: Low FCR rates can indicate that your helpdesk is struggling to resolve issues. Hence, AI can assist by ensuring that more problems are resolved upon first contact directly or by supporting human agents with real-time suggestions.
- Customer feedback: Review customer feedback and satisfaction scores to identify areas for improvement.
Step 3: Select the Best-Fit AI Solution
Here’s a structured approach to guide this process:
Compatibility with existing infrastructure
The AI solution should seamlessly integrate with your existing helpdesk software, CRM systems, and any other tools to ensure a smooth workflow and data consistency. Also, look for solutions that offer customization options for AI’s responses, processes, and learning capabilities.
The AI solution must also be able to scale to respond to your business growth and the corresponding increase in support volume without compromising performance.
Compliance and security
Ensure the AI solution complies with relevant data protection regulations (such as GDPR) and follows best practices in data security to protect sensitive customer information.
Vendor reputation and support
Consider vendors with proven experience in delivering practical AI solutions for helpdesks. Client testimonials, case studies, and independent reviews can provide valuable insights. To ensure long-term success, ensure adequate support for troubleshooting, updates, and maintenance.
Step 4: Prepare the Data
The quality and relevance of the data directly influence the effectiveness of the AI model. This process involves collecting, cleaning, and structuring data that the AI system will use for training. Key components of this phase are:
Data collection
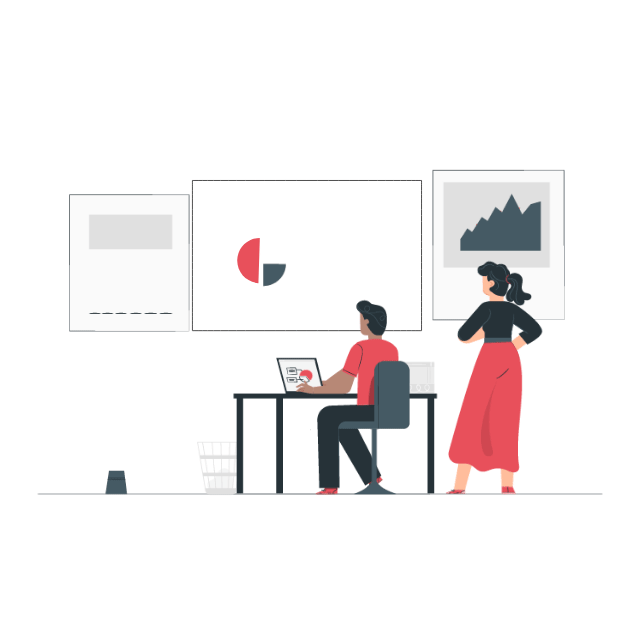
Gather data from past customer interactions across all channels, including email, chat, and phone conversations. This historical data provides the raw material for training the AI model. Plus, utilize CRM records to include customer preferences while incorporating customer feedback to understand common issues, sentiments, and areas for improvement.
Data cleaning and preprocessing
Eliminate any data that is not relevant to training the AI, such as personal information not directly related to customer inquiries. Then, ensure all data is in a uniform format, making it easier for the AI model to process and learn from it. It is also important to address gaps in the data, either by filling in missing values where possible or removing incomplete records to maintain the quality of the training dataset.
Data annotation
Manually tag conversations with relevant labels, such as inquiry type or customer sentiment. Then, annotate parts of the data to highlight customer intent, helping the AI to understand what customers are looking to achieve through their inquiries.
In this step, it’s also important to adopt new technologies like NLP and ML to make the data preparation stage faster, simpler, and more streamlined.
Step 5: Train the Model
Model training is where the AI system learns to understand and respond to customer inquiries based on the data prepared in the previous step. It helps develop an AI model that accurately reflects your helpdesk’s needs. Here’s a breakdown of the model training process:
Selection of ML algorithms
Choose machine learning algorithms best suited for natural language processing tasks, such as deep learning models. These models excel in understanding context, intent, and the subtleties of human language. Also, tailor algorithms to handle specific helpdesk tasks, such as intent recognition, sentiment analysis, and automated response generation.
Training the dataset utilization
Input the cleaned and annotated dataset into the AI model. This data serves as the foundation for teaching the AI about your helpdesk operations, including how to interpret different types of inquiries and the appropriate ways to respond. Additionally, ensure the dataset is balanced across different inquiry types and outcomes to prevent biases in the AI’s responses and decision-making.
Fine-tuning the model
Adjust the model’s hyperparameters (e.g., learning rate, number of layers, etc.) to improve performance and accuracy based on training outcomes. Next, incorporate feedback mechanisms to allow the model to learn from its mistakes and successes, continuously refining its understanding and responses.
Measuring the evaluation metrics
Measure the model’s accuracy in understanding inquiries and providing correct responses. After all, precision in recognizing intent and sentiment is critical. Then, evaluate the AI’s speed in generating responses, aiming for a balance between quick and accurate replies.
Enabling iterative training
AI model training is not a one-time event. Regularly update the model with new data to keep it current with evolving customer needs and organizational changes. Also, employ A/B testing to compare different versions of the AI model, identifying which configurations yield the best outcomes.
Step 6: Testing and Validation
Once the AI model has been trained, the next crucial phase is testing and validation. This step ensures that the model performs as expected, accurately understanding and responding to customer inquiries before it goes live. The process involves:
Performance evaluation
Measure how well the AI model identifies intents and provides relevant responses. It involves comparing the AI’s answers to a set of pre-defined correct answers for a diverse set of queries. Also, assess the AI system’s ability to handle large volumes of inquiries simultaneously without significant drops in performance or speed.
Issue handling
Common issues to watch for include limited scalability, faulty emotion detection, weak ambiguity handling, etc.
Usability testing
Conduct usability tests with real users or helpdesk agents to gather feedback on the AI’s interactions, ensuring they are coherent, contextually appropriate, and add value to the customer experience. Then, ensure the AI is seamlessly integrated into the helpdesk interface, with agents able to use it effortlessly during their interactions with customers.
Iterative improvement
Put in place an intelligent feedback loop to continuously refine the AI based on agent and customer feedback. It includes updating the training dataset with new inquiries and outcomes to improve the model’s accuracy and relevance.
Compliance and security testing
Verify that the AI system complies with data protection regulations, ensuring customer data is handled securely and privacy is maintained. Finally, conduct security assessments to identify any vulnerability in the AI system that could be exploited, ensuring the integrity of the helpdesk operations.
Related article: 20 Best Helpdesk Ticketing Systems & Tools in 2024