Network security is a major focus area for organizations across the globe. With cyber threats becoming more sophisticated, traditional security systems are struggling to keep up. Anomaly detection has emerged as a crucial component in the security infrastructure, serving as an early warning system against unusual patterns that could indicate a security breach. The integration of artificial intelligence (AI) into anomaly detection has significantly enhanced the capability of these systems to identify and respond to threats.
Related blog: AI-Driven Sentiment Analysis: Embracing the Future of Insights
The need for AI in anomaly detection
Traditional network security systems are rule-based, which means they rely on predefined patterns and behaviors to identify threats. However, modern cyber-attacks often deviate from these patterns, making them difficult to detect with rule-based systems alone.
Predefined rules do not constrain AI-driven anomaly detection systems and can learn from data to identify potentially harmful anomalies in network traffic.
AI-driven anomaly detection systems offer several key enhancements that address these issues:
Adaptive learning
AI systems learn from the data they process, enabling them to adapt to new patterns of network behavior and detect anomalies that do not fit previous models. The use of unsupervised learning allows AI to identify threats without prior knowledge of their patterns.
Behavioral analysis
Instead of relying on known signatures, AI systems analyze the behavior of network traffic and can flag activities that, while not matching known threats, are suspicious and merit investigation. This behavioral approach means that AI-driven systems can detect anomalies based on a deviation from baseline behaviors, which is a practical defense against sophisticated, multi-stage attacks.
Scalability and performance
AI systems can handle vast amounts of data and can scale as network traffic grows without a corresponding increase in resources or lag in performance. They can operate continuously and autonomously, providing round-the-clock monitoring without the need for breaks or downtime.
Advanced pattern recognition
Machine learning, especially deep learning, excels at identifying complex patterns within data that are not discernible to human analysts or traditional systems. These capabilities are crucial for detecting advanced persistent threats (APTs) that reside within networks for long periods and aim to avoid detection.
How AI-powered anomaly detection work?
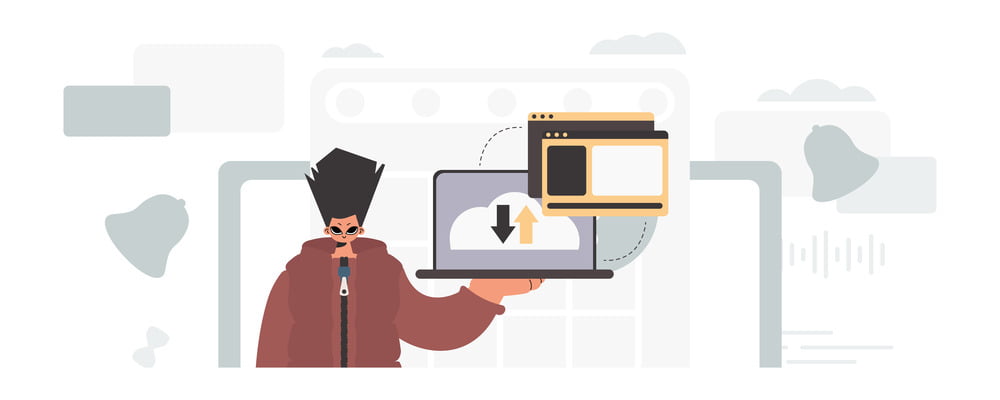
AI-driven anomaly detection uses machine learning algorithms to process large volumes of network data and learn from it. These systems become smarter by harnessing historical data to understand what normal network behavior looks like. Once the learning phase is complete, the AI system can then monitor network traffic in real-time to identify deviations from the norm.
Machine learning models used in anomaly detection can be categorized into three types:
- Supervised Learning: These models are trained on labeled data (normal and abnormal) and are sufficient when you have a dataset that contains known anomalies.
- Unsupervised Learning: These models work with unlabeled data and are useful when you do not have examples of anomalies. They detect anomalies by looking for data points that differ significantly from the majority.
- Semi-Supervised Learning: These models are trained on a dataset that is mostly labeled as ‘normal.’ They learn the ‘normal’ behavior and flag deviations as potential anomalies.
Deep learning has also proven particularly useful for anomaly detection. Neural networks, with their ability to detect complex patterns and relationships in data, are excellent at identifying subtle anomalies that might escape other methods.
Benefits of AI-driven anomaly detection
Enhanced Detection Accuracy: AI algorithms, particularly those using machine learning, can analyze vast datasets to identify patterns and anomalies with greater accuracy than traditional systems. It reduces the rate of false positives and negatives, allowing security teams to focus on genuine threats.
Real-Time Threat Identification: AI-driven systems can process and analyze data in real-time, providing immediate alerts when potential security incidents are detected. This timely response is crucial for preventing damage that could result from delayed detection.
Cost-effectiveness: Although there is an initial investment in setting up AI-driven anomaly detection, over time, these systems can be cost-effective by automating the detection process, reducing the workload on human analysts, and minimizing the costs associated with security breaches.
Proactive security posture: AI-driven systems can identify subtle, emergent patterns that suggest a threat before it fully materializes. This proactive approach can help in preempting attacks, thereby shifting the security strategy from reactive to anticipatory.
Reduction in incident response time: When an anomaly is detected, AI-driven systems can initiate automated responses to contain the threat. This rapid response capability can significantly reduce the time it takes to mitigate a security incident, limiting potential damage.
Advanced analytics and insights: AI anomaly detection systems can provide deeper insights into network behaviors, creating opportunities for improving network design and policy. These analytics can help in refining security protocols and in making informed decisions about network and security architecture.
Scalability: As network traffic volume grows, AI systems can scale accordingly without a loss in performance. They can handle increased data loads, making them suitable for large and expanding network environments.
Comprehensive coverage: AI-driven anomaly detection doesn’t just look for known threats but also uncovers new, previously unidentified threats. This comprehensive coverage is vital in a landscape where attackers constantly devise new methods to breach networks.
Related blog: How Generative AI is Reshaping the ITOps Landscape?
Future direction
While AI-driven anomaly detection offers numerous benefits, there are challenges in its implementation. For instance, the complexity of AI models requires skilled personnel to manage and interpret the results. These systems also require access to large volumes of high-quality data to learn. Privacy is another concern, as the data used to train AI systems may contain sensitive information that must be protected.
However, as AI continues to evolve, the potential for anomaly detection systems will increase. For example, advancements in unsupervised and semi-supervised learning algorithms are particularly promising. They reduce the need for labeled data, which can be costly and time-consuming to produce. So, despite the challenges, the implementation of AI in network security systems is a critical step towards a more secure digital environment for businesses and individuals alike.