As a sales and marketing specialist, you always strive to provide a seamless and personalized experience for your customers and prospects. However, it can be challenging to thoroughly understand their opinions and emotions during a conversation and over time when they interact with your business regularly. This is particularly true if you connect with them mainly through online platforms like social networking, online reviews, and support interactions.
And do you know 86% of buyers are willing to pay more for excellent customer experience (CX)? You probably catch our drift – that CX will be the competitive difference for most brands going forward. So, it is essential to provide exceptional customer experience to stay ahead of competitors! But how do you analyze your customer opinions and feedback?
Fortunately, AI-driven sentiment analysis can assist you in better understanding and engaging with your customers so that you can improve the quality of your customer interactions, boost your sales rate, and optimize conversation performance.
Related blog: AI for Customer Support and Why You Need It
Ready to learn more about AI-powered sentiment analysis? Let’s dive in!
AI-Driven Sentiment Analysis: A Game Changer
Do you know artificial neural networks were able to reach 85% accuracy in understanding the sentiment of text analysis?
Talking to your customers can help you gain a deeper understanding of how they feel about your brand and can also provide you with market trends and development opportunities. In today’s digital era, customers frequently express their opinions about businesses through a variety of channels. After all, there is a huge amount of unstructured data to manage. Using AI-driven sentiment analysis, you can fetch insights from vast volumes of customer data quickly and stay on top of what customers are saying about your brand.
Sentiment analysis, often termed opinion mining, is an approach for identifying and assessing the sentiments present in a text. The main goal of this analysis is to determine whether a specific topic, product, or service is seen positively, negatively, or neutrally. This process utilizes machine learning and natural language processing (NLP) to transform how businesses communicate with customers. Sentiment analysis allows businesses to go beyond traditional count-based analytics and gain high-value insights from social media streams and consumer conversations. As a result, companies are better able to understand their customers’ sentiments and ultimately improve the products and services they offer.
How Sentiment Analysis with AI Works?
Sentiment analysis with AI involves a combination of linguistic analysis, machine learning techniques, and computational algorithms to discern and quantify emotions within text data. It’s a powerful tool for understanding public opinion, customer feedback, and market trends, which can inform decision-making for businesses and organizations.
Here’s a simplified explanation of how it works:
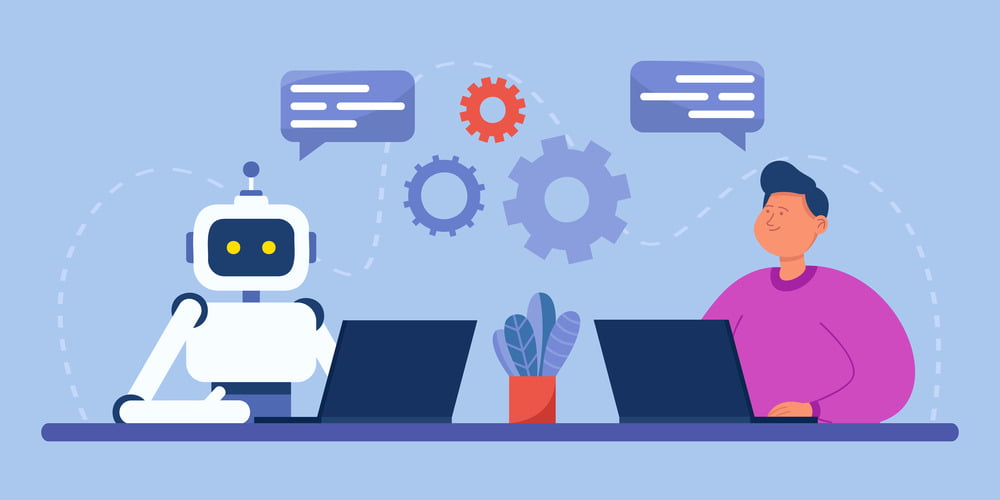
Data Collection
The process begins with gathering textual data from various sources like social media platforms, customer reviews, surveys, or any text-containing documents relevant to the analysis.
Pre-processing
Before analysis, the text data undergoes pre-processing steps. This includes tasks like removing punctuation, converting all text to lowercase, and handling special characters or symbols.
Tokenization
The text is then broken down into smaller units called tokens. Tokens are typically words or groups of words. For example, the sentence “I love this product!” would be tokenized into [“I”, “love”, “this”, “product”, “!”].
Feature Extraction
In this step, the relevant features or attributes are identified from the tokens. This could involve techniques like Bag-of-Words (BoW) or Term Frequency-Inverse Document Frequency (TF-IDF) to represent the text in a numerical format that can be understood by the AI model.
Training the Model
This is a crucial step where the AI model is trained using labeled data. Labeled data means that each piece of text is associated with a sentiment label (e.g., positive, negative, neutral). The model learns to identify patterns in the data that correspond to different sentiments.
Model Evaluation and Fine-Tuning
After training, the model’s performance is evaluated using a separate set of data (validation data) that it hasn’t seen before. If the model doesn’t perform well, it may be fine-tuned by adjusting parameters or using more complex architectures.
Predicting Sentiment
Once the model is trained and validated, it can be used to predict sentiment in new, unseen text. The model assigns a sentiment label (e.g., positive, negative, neutral) based on the patterns it has learned during training.
Post-processing and Interpretation
The output of the model may go through post-processing steps, like converting numerical scores into human-understandable sentiment labels. The results are then interpreted for actionable insights.
Feedback Loop (Optional)
The model’s performance can be continuously improved by incorporating user feedback. If the model makes incorrect predictions, this feedback can be used to retrain and enhance its accuracy.
Related blog: Conversational AI Service Desk: The Future of ITSM
Significance of Sentiment Analysis Tool
A sentiment analysis tool is software that analyses text exchanges and evaluates the tone, emotion, and intent of each message. The software delves further into these elements to provide more context from your conversations and helps your customer service team appropriately analyze comments. If your company delivers an omnichannel experience, a sentiment analysis tool might save your team time organizing and reporting customer comments.
Rather than going through each tweet and comment one by one, a sentiment analysis tool analyses your feedback and determines whether it is positive, negative, or neutral. The information is then integrated and displayed in graphs or charts that clearly show the patterns in your customers’ comments. In addition to giving your team specific information to work with, doing this gives your team extra time to perform other tasks as part of their usual workflow.
Top AI Sentiment Analysis Tools
Prior to now, sentiment analysis required the study of hundreds of comments, postings, and survey results. Today, you can use one of the many sentiment analysis tools available to automate the procedure and provide your team with the information they need to succeed.
Let’s look more closely at the list of the top sentiment analysis tools:
OpenText
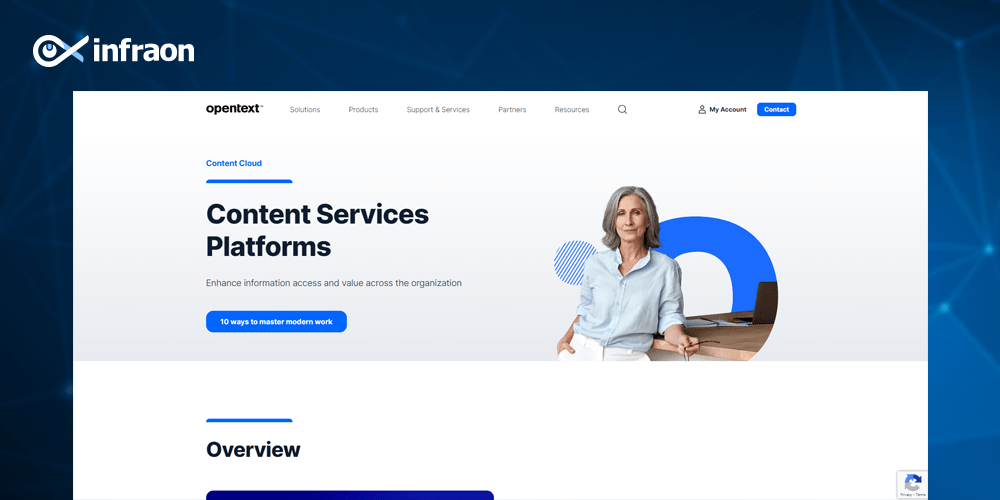
The OpenText Content Analytics platform is a comprehensive solution designed to extract valuable insights from large volumes of textual data. A notable feature within this platform is the Sentiment Analysis Tool. This tool is specifically tailored to identify and categorize emotional expressions and irrational patterns within text. This capability is invaluable for businesses looking to gauge the sentiment and emotional tone present in various types of textual content. By employing this tool, users can gain a deeper understanding of customer feedback, social media conversations, and other text-based data sources.
Brand24
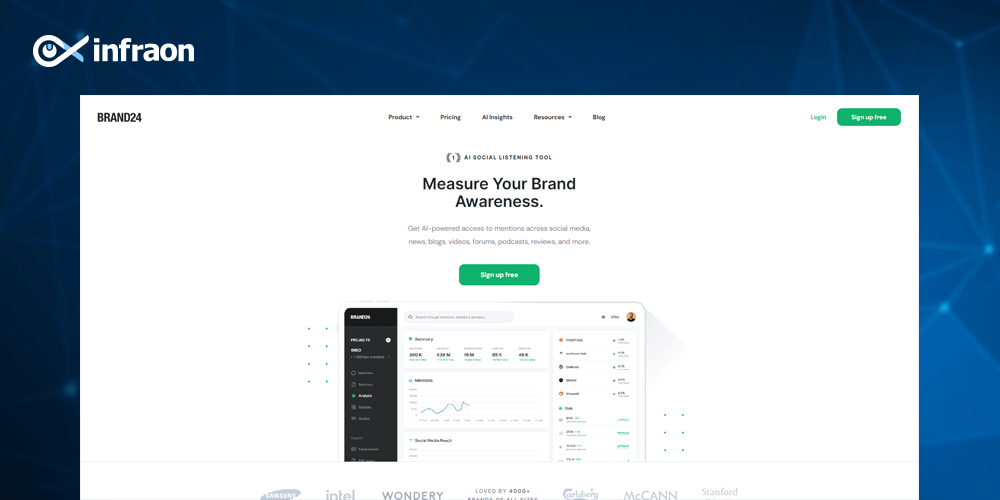
Brand24 is a robust media monitoring tool that offers a range of functionalities, with sentiment analysis being one of its key features. It specializes in tracking and monitoring online mentions across a wide array of sources, including social media networks, blogs, forums, websites, podcasts, and newsletters. This tool provides businesses with a comprehensive view of how their brand, products, or keywords are being discussed online. Through sentiment analysis, Brand24 allows users to assess the prevailing sentiment associated with their brand, providing valuable insights into public perception.
ParallelDots
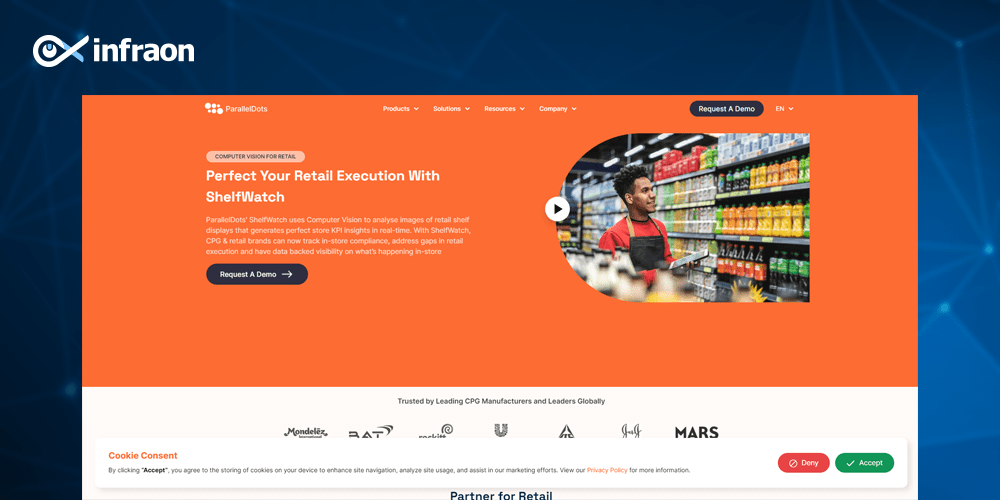
ParallelDots is an applied AI research team that offers a suite of AI-powered services, with sentiment analysis being one of their offerings. Their sentiment analysis product is accessible through APIs, enabling seamless integration into various applications and workflows. ParallelDots categorizes its offerings into Products, APIs, and Plugins, with sentiment analysis forming a part of its comprehensive AI portfolio.
Lexalytics
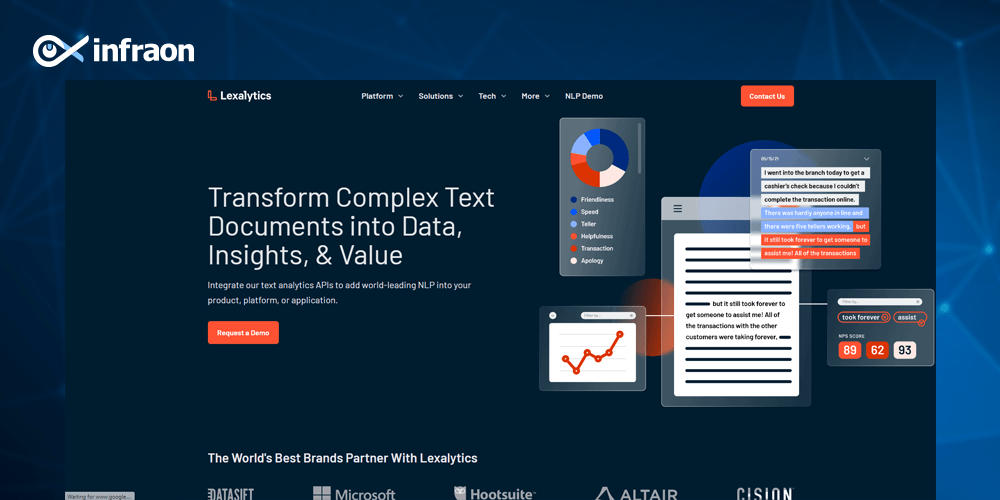
Lexalytics is a versatile text analytics platform that caters to organizations dealing with substantial volumes of textual data. It encompasses a range of features, including the ability to process text data at scale. Moreover, Lexalytics provides the option for secure on-premise deployment, ensuring that sensitive data remains within the organization’s infrastructure. This tool empowers users to fine-tune and customize their text analytics to suit their specific needs.
Hi-Tech BPO
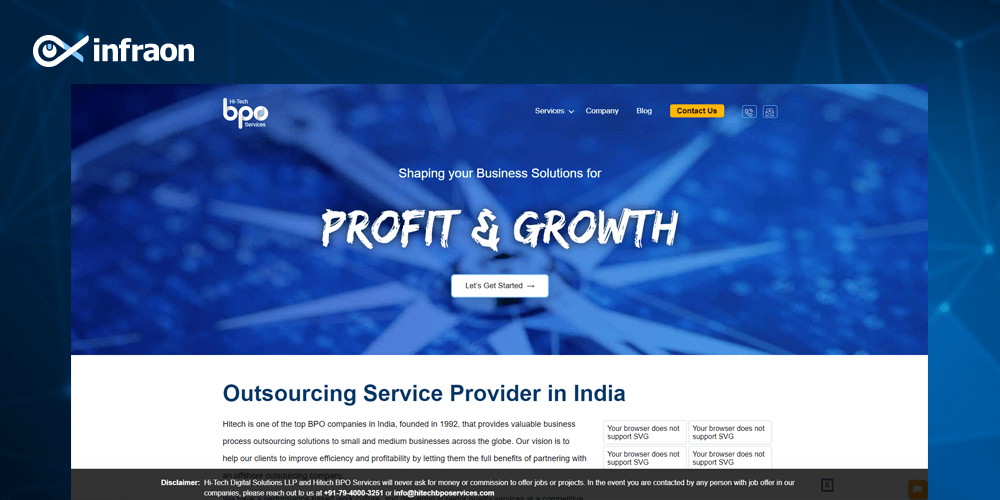
Hi-Tech BPO offers a robust sentiment analysis program as part of its suite of services. In addition to sentiment analysis, the company provides services spanning data analytics, market intelligence, and data processing. One of the distinctive strengths of Hi-Tech BPO is its proficiency in deciphering the meaning behind product and service reviews presented in various formats, including text, audio, emoji, photos, and other visual mediums. This comprehensive approach enables businesses to extract valuable insights from a wide range of customer feedback channels.
Social Mention
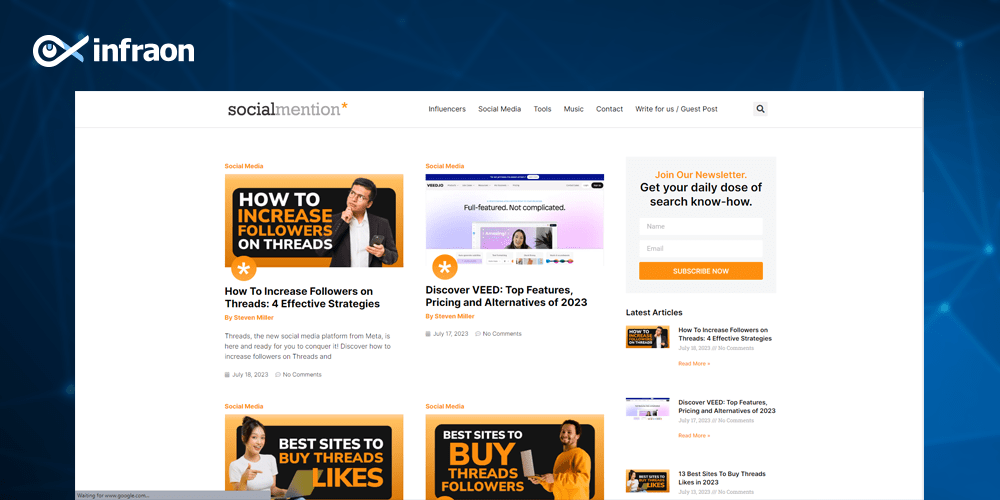
Social Mention is one of the free social media search engine tools that aggregate user-generated content from various social media platforms. It casts a wide net by collecting information from platforms such as Reddit, Flickr, and Google News. Additionally, Social Mention offers Twitter sentiment analysis. This tool provides users with a consolidated view of how their brand or keywords are being discussed across diverse social media channels.
Social Searcher
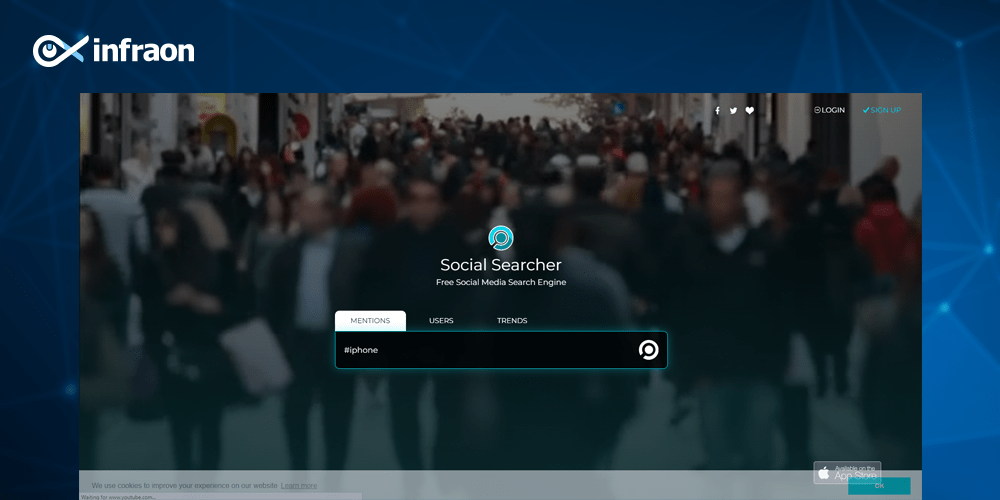
Social Searcher is a comprehensive product that offers a range of features, including Social Buzz, Google Social Search, and Media Monitoring. While it can be used without signing up, there are distinct advantages to creating an account. This tool provides an extensive suite of functionalities for monitoring and analyzing social media content, enabling businesses to stay informed about their online presence and reputation.
Sentiment Analyzer
Sentiment Analyzer is a free tool designed for analyzing English-language text. It employs a scale ranging from -100 to +100, where -100 represents a negative sentiment, and +100 indicates a positive sentiment. Users must manually input the text they wish to analyze, as it does not automatically retrieve data. The tool was constructed using a dataset of nearly 8,000 written examples of spoken interactions, ensuring its effectiveness in assessing sentiment in various contexts.
Meaning Cloud
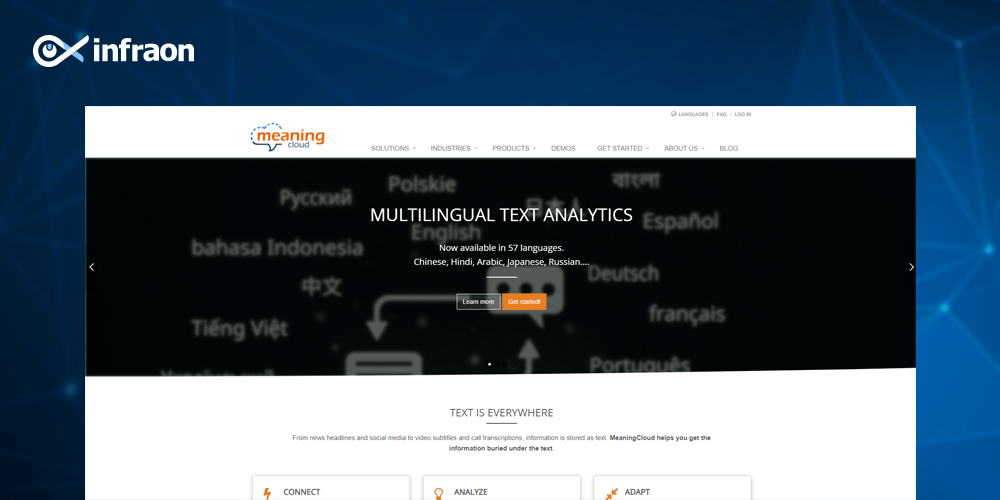
Meaning Cloud offers a wide range of products, one of which is its sentiment analysis tool. This tool operates through an API that analyzes text by identifying specific phrases and assessing their relationships to one another. This approach allows for a nuanced understanding of sentiment within textual content, enabling businesses to extract valuable insights from their data.
Related blog: Why ITSM needs the human touch in the age of AI
Understanding the Use Cases of AI-Driven Sentiment Analysis
Sentiment analysis is a robust process that allows organizations to derive insights from unstructured data and reinvent business strategies that are most compelling to the target market. Let’s see some of its use cases:
Social Media Monitoring
In the realm of social media, businesses utilize sentiment analysis to gain a deep understanding of how people perceive their brands and products. Here’s how it works:
- Brand Perception: Sentiment analysis helps businesses gauge whether the sentiment towards their brand is positive, negative, or neutral in social media conversations. This can involve tracking hashtags, mentions, and comments related to their products or services.
- Competitor Analysis: Companies can also use sentiment analysis to monitor the sentiment around their competitors. By analyzing social media discussions and customer sentiment towards rival brands, businesses can identify areas where they can outperform the competition.
- Trend Tracking: Sentiment analysis allows businesses to identify emerging trends and topics in social media discussions. This information is valuable for staying ahead in the market and creating content that resonates with the current social media climate.
- Crisis Management: Real-time sentiment analysis can be crucial during a crisis or viral event. It helps in identifying potential PR crises early on, enabling businesses to respond promptly and effectively. For instance, airlines can monitor social media sentiment during flight delays to address passenger concerns promptly.
Customer Feedback and Reviews
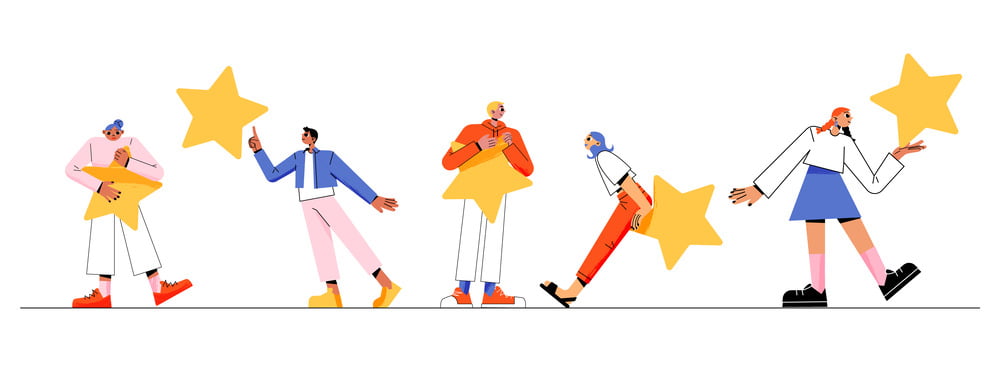
Sentiment analysis is a game-changer when it comes to processing and understanding customer feedback and reviews:
- Automated Categorization: Companies can use sentiment analysis to automatically categorize customer reviews into positive, negative, or neutral sentiments. This streamlines the process of analyzing large volumes of feedback, saving time and resources.
- Strengths and Weaknesses: By analyzing sentiment in customer reviews, businesses can pinpoint the specific strengths and weaknesses of their products or services. For example, a restaurant chain can identify which menu items are receiving positive feedback and which need improvement.
- Product Improvement: Sentiment analysis helps businesses identify areas for product or service improvement. When negative sentiments are consistently associated with particular features or aspects, companies can focus their efforts on addressing those issues.
Market Research
Sentiment analysis plays a pivotal role in market research:
- Consumer Preferences: By analyzing sentiments expressed in discussions, comments, and reviews, companies can gain insights into consumer preferences and sentiments about their products or industry trends.
- Competitor Analysis: Businesses can also use sentiment analysis to monitor consumer sentiment towards competitors’ products and services. This information is valuable for identifying gaps in the market and potential opportunities for differentiation.
- Product Development: Sentiment analysis informs product development by highlighting areas where customers are dissatisfied or where there is strong demand. This data-driven approach ensures that new products align with customer needs and desires.
Brand Reputation Management
Managing brand reputation is essential for long-term success, and sentiment analysis plays a key role:
- Monitoring Online Conversations: Sentiment analysis tools continuously monitor online conversations, news articles, blogs, and social media mentions related to the brand. This allows companies to stay vigilant and respond to any negative sentiment promptly.
- Mitigating Damage: When negative sentiment arises, companies can address it proactively. For instance, a hotel chain can respond to a negative review on social media by offering a solution to the customer’s problem, showcasing their commitment to customer satisfaction.
- Identifying Improvement Areas: Sentiment analysis not only helps in addressing immediate concerns but also in identifying recurring issues or areas for improvement in products, services, or customer interactions.
Customer Support and Feedback Management
Integrating sentiment analysis into customer support systems transforms how companies handle customer inquiries and feedback:
- Automated Ticket Prioritization: Sentiment analysis automatically categorizes and prioritizes support tickets based on the sentiment expressed by customers. Urgent issues or highly negative feedback can be addressed with higher priority, ensuring better customer satisfaction.
- Efficiency: Customer support teams can work more efficiently as they can focus their attention on high-impact issues rather than manually sorting through a large volume of requests.
- Proactive Problem Solving: Sentiment analysis helps in proactively identifying customer problems or concerns, even before customers explicitly express them. This enables companies to resolve issues before they escalate.
Final Note
AI makes sentiment analysis faster and more accurate. It gives quick feedback on how customers are feeling about your brand. However, when applying AI for sentiment analysis, there are many ethical issues that must be taken into account, such as bias, privacy, and transparency.
Looking to the future, there will be more exciting developments and trends in AI for sentiment analysis, including NLP, explainable AI, multimodal analysis, contextual analysis, and real-time analysis. By investing in the latest AI technologies and staying up-to-date with these emerging trends, businesses can gain a competitive edge and provide a greater level of customer experience.